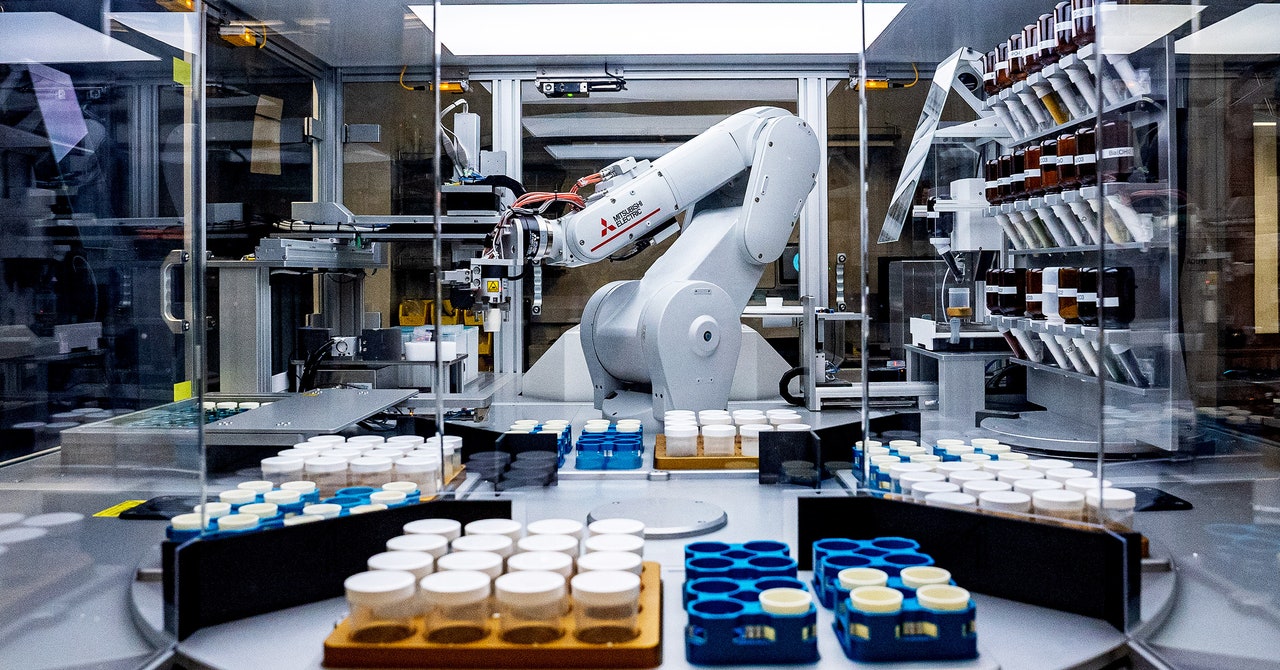
The robotic line cooks were deep in their recipe, toiling away in a room tightly packed with equipment. In one corner, an articulated arm selected and mixed ingredients, while another slid back and forth on a fixed track, working the ovens. A third was on plating duty, carefully shaking the contents of a crucible onto a dish. Gerbrand Ceder, a materials scientist at Lawrence Berkeley Lab and UC Berkeley, nodded approvingly as a robotic arm delicately pinched and capped an empty plastic vial—an especially tricky task, and one of his favorites to observe. “These guys can work all night,” Ceder said, giving two of his grad students a wry look.
Stocked with ingredients like nickel oxide and lithium carbonate, the facility, called the A-Lab, is designed to make new and interesting materials, especially ones that might be useful for future battery designs. The results can be unpredictable. Even a human scientist usually gets a new recipe wrong the first time. So sometimes the robots produce a beautiful powder. Other times it’s a melted gluey mess, or it all evaporates and there’s nothing left. “At that point, the humans would have to make a decision: What do I do now?” Ceder says.
The robots are meant to do the same. They analyze what they’ve made, adjust the recipe, and try again. And again. And again. “You give them some recipes in the morning and when you come back home you might have a nice new soufflé,” says materials scientist Kristin Persson, Ceder’s close collaborator at LBL (and also spouse). Or you might just return to a burned-up mess. “But at least tomorrow they’ll make a much better soufflé.”
Video: Marilyn Sargent/Berkeley Lab
Recently, the range of dishes available to Ceder’s robots has grown exponentially, thanks to an AI program developed by Google DeepMind. Called GNoME, the software was trained using data from the Materials Project, a free-to-use database of 150,000 known materials overseen by Persson. Using that information, the AI system came up with designs for 2.2 million new crystals, of which 380,000 were predicted to be stable—not likely to decompose or explode, and thus the most plausible candidates for synthesis in a lab—expanding the range of known stable materials nearly 10-fold. In a paper published today in Nature, the authors write that the next solid-state electrolyte, or solar cell materials, or high-temperature superconductor, could hide within this expanded database.
Finding those needles in the haystack starts off with actually making them, which is all the more reason to work quickly and through the night. In a recent set of experiments at LBL, also published today in Nature, Ceder’s autonomous lab was able to create 41 of GNoME’s theorized materials over 17 days, helping to validate both the AI model and the lab’s robotic techniques.
When deciding if a material can actually be made, whether by human hands or robot arms, among the first questions to ask is whether it is stable. Generally, that means that its collection of atoms are arranged into the lowest possible energy state. Otherwise, the crystal will want to become something else. For thousands of years, people have steadily added to the roster of stable materials, initially by observing those found in nature or discovering them through basic chemical intuition or accidents. More recently, candidates have been designed with computers.
The problem, according to Persson, is bias: Over time, that collective knowledge has come to favor certain familiar structures and elements. Materials scientists call this the “Edison effect,” referring to his rapid trial-and-error quest to deliver a lightbulb filament, testing thousands of types of carbon before arriving at a variety derived from bamboo. It took another decade for a Hungarian group to come up with tungsten. “He was limited by his knowledge,” Persson says. “He was biased, he was convinced.”